Now more than ever, enterprises are always on the lookout for new ways to implement artificial intelligence algorithms across various sectors as they strive to remain competitive. AI is changing the way businesses operate, from automating routine tasks to making data-driven decisions like in the example of the Digital Twin. On the other hand, the growing scale of these services, infrastructure components, and performance monitoring tools is also leading to an increase in the complexity of their deployments and the generation of large volumes of data. This is where artificial intelligence for IT Operations comes into the picture. The term, coined by Gartner in 2016 describes AIOps as combining big data and machine learning to automate IT Operations processes, including event correlation, anomaly detection, and causality determination1. In the current technological environment, where high availability, scalability, and efficiency are imperative, AI-powered implementation, management, and delivery of IT Services are essential to achieving these goals.
AIOps explained
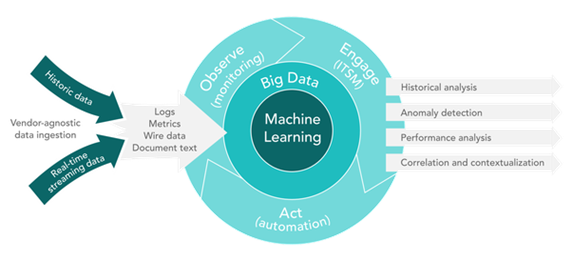
As previously mentioned, in order to support and streamline IT Operations, an AIOps platform needs to collect, ingest and analyse an ever-increasing variety and volume of data from diverse sources. This is why the 3 defining features of AIOps can be summarized as follows:
- Big Data aggregation and analysis
As with all AI Models, AIOps platforms need huge amounts of data to ensure consistency and train the algorithms responsible for making accurate predictions and decisions. This data can come in various forms, including error logs, performance numbers, metrics, traces, and other technical details from enterprise technology setups such as applications, servers, and networks.
- Machine learning algorithms
Machine learning plays a crucial role in AIOps as it’s responsible for processing and analysing historical data as a means to spot patterns, identify application performance issues and detect anomalies. Through its use, AIOps platforms can achieve full automation of tasks and processes, which usually require human intervention.
- Automation
Automating resolutions of time-heavy issues and processes and coordinating those across multiple IT Systems reduces the workload for IT operators and allows them to focus on more strategic initiatives and enhance their efficiency. For example, automating processes like data backups, anomaly detection and root cause analysis helps prevent potential problems before they have even impacted the system or take the needed corrective actions, ensuring higher availability and reliability.
Differences and Similarities: AIOps and DevOps
As technical architecture, requirements and practices evolve, a wide range of new terms are emerging to meet them, often confusing because of how similar they look and sound. One such example is AIOps and DevOps – two distinct yet interconnected methodologies, each addressing specific aspects and complementing each other to drive continuous improvement in modern enterprises.
Though both can involve the use of machine learning and other artificial intelligence technologies, AIOps primarily deal with the infrastructure and operations side, while DevOps is used within the software development and delivery lifecycle for a vast range of processes, including automated testing, continuous integration and deployment, code quality assessment and improving resource management.
Despite this distinction, the two can interact with each other to bring high effectiveness among operations and development teams. For example, AIOps can help DevOps teams identify critical issues in a sea of alerts by aggregating alerts, recognizing patterns and correlation incidents, ensuring noise reduction and optimizing resource allocation. This cross-functional teamwork between software development and IT Operations creates systems that are not only more reliable but also operate with greater efficiency and effectiveness.
Real-world Applications
As previously pointed out, AIOps can support a wide spectrum of IT Operations use cases. Some of the key features that are becoming central to leading enterprises’ strategies include:
- Anomaly Detection
Anomaly detection is the process of identifying outlier events – data points that deviate from historical patterns and could suggest potential problems. The need of implementing AIOps for anomaly detection stems from the massive amount of data volume, generated in large-scale environments with lots of components, each one logging countless rows of data with multiple columns, such as timestamps, hardware, the origin datacentre, etc. Many algorithms can be used to approach this, like focusing on a selected set of metrics expected to behave similarly and raising an alert if any of them differ from the norm. Recently, Microsoft Research and Microsoft Azure introduced a new algorithm for identifying and localizing anomalies in high dimensional time series data, called AiDice, which transformes the anomaly detection process into a combinatorial optimization problem, making it less time consuming and more accurate and sacalable2.
- Event correlation and Root Cause Analysis
Root Cause Analysis is the process of finding the underlying reasons for any anomalies. Through analysing large volumes of data, AIOps can establish what is normal and what’s not concerning the behaviour in the IT environment. It can then connect the dots by finding relationships between data points and looking for similar past scenarios to determine the issue’s potential causes and severity level. Beyond this, it can also predict the potential ripple effects, meaning how one problem might lead to another via dependency mapping and context visualisation, which help track the dependencies and interactions between different components in the infrastructure.
- Automated Remediation
In combination with identifying the underlying causes of incidents, AIOps can help reduce the mean time to resolve (MTTR) by using predictive IT management to automate issue resolution and prevent these incidents before they even occur. There are multiple approaches to implementing automated remediation – for instance, once an anomaly has been detected, the AIOps tool can automatically trigger an incident response and remediation workflow, which can include restarting the service or sending an alert. Another method typically used in cloud environments would be using the ML models to predict what kind of problem is likely to happen at a given time in any component and then automatically scaling the cloud resources up or down and migrating the data to a different region.
- Eliminating tool sprawl
IT Teams often spend a lot of their time mastering a tool only to then have to learn a new tool, which leads to spending a lot of time just managing technologies and infrastructure rather than allocating their time and resources to more productive tasks. This is the so-called IT tool sprawl – managing multiple tools and applications across the IT environment. AIOps offers a solution to this by bringing all IT tools to one centralized place for monitoring and management. Through AI and automation, it can then analyse data from various resources and trigger alerts and remediation processes to get rid of the need for meeting across different company departments.
Case Studies
Yahoo
A real-world example of these benefits is Yahoo’s adoption of AIOps through the Moogsoft platform. By implementing AIOps, Yahoo was able to shift from a complex and highly heterogeneous technology environment to a more streamlined, efficient, and proactive IT Operations management system, as detailed in their case study3.
Being a company built through many acquisitions like Yahoo Finance, Tumblr, and TechCrunch, Yahoo had found it hard navigating through the complex growing environment of legacy code, cloud systems, and various infrastructures. Of course, they aimed at improving this through implementing modern architecture and infrastructure principles, like switching over to AWS and public cloud services, as well as implementing microservices, continuous delivery, and DevOps. Yet, IT operations teams still struggled with the complexity and interdependency of their systems, resulting in an overwhelming amount of noise and difficulty in identifying the underlying root causes of all these alerts. Anytime a breakdown would happen anywhere in the service chain it could trigger thousands of alerts and cause multiple service failures, which was beyond their traditional operations management toolsets’ capacity to handle. Yahoo’s infrastructure could generate about 2 million alerts a day, leaving engineers struggling to discern the significant ones from the noise and wasting a lot of useful time because they couldn’t manage the entire scope of impact.
To address this challenge, Yahoo turned to Moogsoft, an AI-powered platform for DevOps, Site Reliability Engineering and ITOps. Their machine learning algorithms reduced the alert noise by correlating the similar incidents into clusters, providing root cause analysis and enabling faster remediation. This integration helped Yahoo’s operations team manage over 400 business services and internal infrastructure and prevent costly outages, reducing the 2 million alerts down to 10 000, achieving a 99% noise reduction.
APIS IT
Similarly, APIS IT, the central agency for the Croatian government, integrated IBM Instana Observability and IBM Turbonomic solutions to improve their IT operations. This was achieved by maximizing application performance through insights into the status of all critical infrastructure components and applications and smart automatic alerts from Instana, and automatic resourcing recommendations from Turbonomic. With these solutions in place, APIS IT saw an increase in resourcing decisions, up to 50% faster MTTR (mean-time-to-repair) and a 15% proactive incident avoidance, among other benefits.4
Challenges of AIOps
As one might expect, implementing these advanced systems comes with a set of challenges. One example of this is the cultural shift within a company that comes with adopting AIOps, as users have to build trust towards these systems in order to use it to its full capacity, by promoting data-driven decision-making and problem solutions based on the platform’s insights. Another potential cause for internal organizational conflicts comes from the initial cost of implementing these solutions, including the investment in the technology itself, and the training of the involved teams. Moreover, it is often the case that individual teams have their own established set of tools that they don’t want to change, leading companies to keep their existing systems and adding an AIOps platform on top. One other significant hurdle in implementing an AIOps system comes from its reliance on large volumes of data, including company-sensitive or personal data, which can pose various cybersecurity and privacy risks. Of course, there are steps that companies can take to keep up with the most up-to-date data protection regulations, like robust data encryption, rigorous access control, and regular system audits.
The Future of AIOps
“There is no future of IT Operations that does not include AIOps”, as pointed out by Gartner in the 2022 Gartner Market Guide for AIOps Platforms5. Further findings include that AIOps is continuing its growth and influence on the IT Operations market with a projected market size of $2.1 billion by 2025 with an annual growth rate of around 19%. These numbers highlight just how much the demand for digital transformation has grown in the previous years and will continue to increase and as the digital landscape and infrastructure of modern enterprises grows more complex, the integration of machine learning in many solutions, including artificial intelligence for IT Operations will become crucial. Early adopters of this innovation are already seeing its benefits and enterprises would be smart to follow, as AIOps is leading this transformation, making it the ideal tool to maximize efficiency via automation and ensure availability via smart incident prediction, prevention and resolution.
- Gartner. https://www.gartner.com/en/information-technology/glossary/aiops-platform ↩︎
- Russinovich, Mark (2022, 3.10). Advancing anomaly detection with AIOps—introducing AiDice, https://azure.microsoft.com/en-us/blog/advancing-anomaly-detection-with-aiops-introducing-aidice/ ↩︎
- Yahoo: From Alert Fatigue to Actionable Operational Insights, (2023, 01.01). https://www.moogsoft.com/case-studies/yahoo-alert-fatigue-actionable-operational-insights/ ↩︎
- Maximizing performance of critical government services. IBM. https://www.ibm.com/case-studies/apis-it-aiops ↩︎
- Gartner. https://www.gartner.com/en/documents/4015085 ↩︎
Leave a Reply
You must be logged in to post a comment.